MACHINE LEARNING /
ARTIFICIAL INTELLIGENCE
WE EVALUATE ML/AI ON THREE AXES
TECHNICAL ABILITY
How well does the tech-as-built and the tech team measure up against industry norms?
We’ll look at if they using the right tools, leveraging the right data, guarding against bias and creep, can measure and defend their accuracy claims, and rapidly get new models out of R&D and into profit-making production.
BUSINESS ALIGNMENT
How relevant is the ML/AI to their overall value?
Does the ML/AI represent significant competitive value? How replicable is it by existing competitors or new entrants? What strategic disconnects do we see between target’s stated ML/AI goals and their ML/AI effort to date? Does ML/AI have a seat at the product/strategy table or just told what to do?
BLIND SPOTS
How can the ML/AI be improved? Both quickly and for longer term competitive advantage?
Is there low hanging fruit and what are the time frames for quick improvements to ROI, stickiness, revenue, profit and/or widen competitive moats? What are longer term improvements? Is new talent needed? Does the data science team see and understand where the business value is?

A TALE OF TWO TIMELINES
ML/AI Before Technology Due Diligence
For some deals, the main value driver is the ML/AI. For such deals, it’s not worth looking beyond ML/AI unless and until the ML/AI checks out. We often review ML/AI up front and tell you if there’s enough “there there” to warrant continued interest.
Phase I answers usually available within 8 days and does not require source code access.
ML/AI During Full Technology Due Diligence
For most deals, ML/AI is simply part of technology due diligence along with other areas like security, scalability, open source, etc…
Note: ML/AI often implicates privacy issues under the GDPR and HIPAA, which can sometimes undermine or challenge certain business models. We review privacy regime constraints during full technology due diligence.
Case Studies
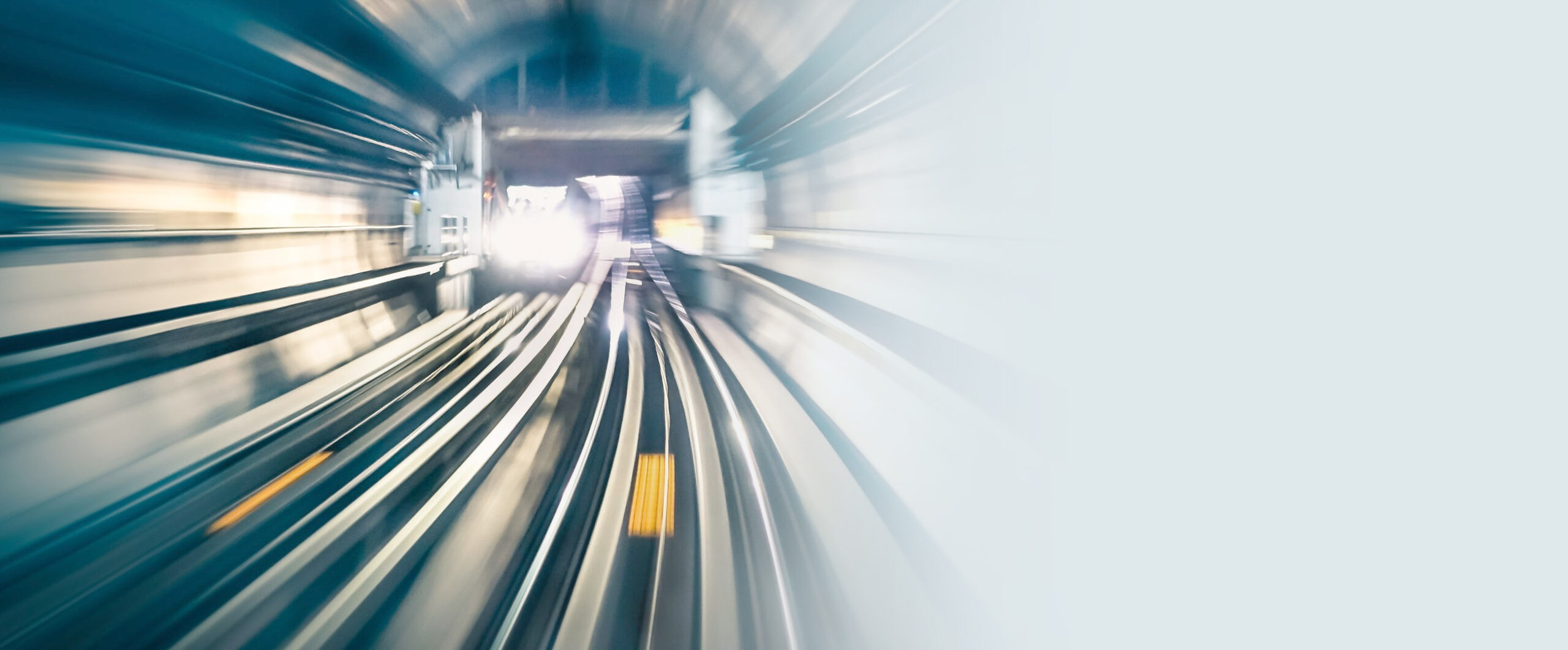